import hamux as hmx
from hamux.lagrangians import lagr_identity, lagr_sigmoid, lagr_softmax, lagr_tanh
import jax
import jax.numpy as jnp
import jax.random as jr
import jax.tree_util as jtu
import equinox as eqx
HAMUX
What is HAMUX?
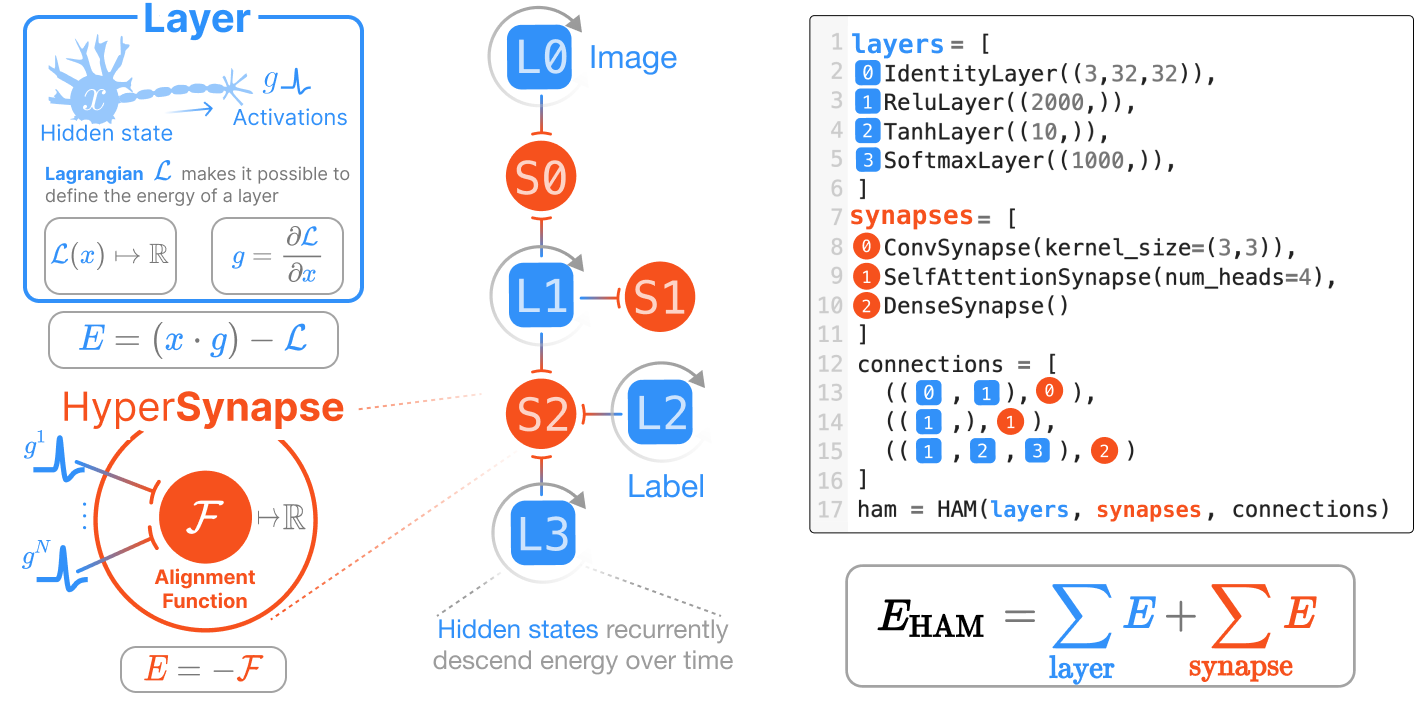
HAMUX defines two fundamental building blocks of energy: the 🌀neuron layer and the 🤝hypersynapse (an abstraction of a pairwise synapse to include many-body interactions) connected via a hypergraph. It is a fully dynamical system, where the “hidden state” \(x_i^\ell\) of each layer \(\ell\) (blue squares in the figure below) is an independent variable that evolves over time. The update rule of each layer is entirely local: neurons evolve deterministically by accumulating “signals” from only the connected synapses (i.e., the red circles in the figure below). This is shown in the following equation:
\[\tau \frac{d x_{i}^{\ell}}{dt} = -\frac{\partial E}{\partial g_i^\ell}\]
where \(g_i^\ell\) are the activations (i.e., non-linearities) on each neuron layer \(\ell\), described in the section on Neuron Layers. Concretely, we implement the above differential equation as the following discretized equation (where the bold \({\mathbf x}_\ell\) is the collection of all elements in layer \(\ell\)’s state):
\[ \mathbf{x}_\ell^{(t+1)} = \mathbf{x}_\ell^{(t)} - \frac{dt}{\tau} \nabla_{\mathbf{g}_\ell}E(t)\]
HAMUX handles all the complexity of scaling this fundamental update equation to many 🌀neurons and 🤝hypersynapses with as minimal overhead as possible. Essentially, HAMUX is a simplified hypergraph library that allows us to modularly compose energy functions. HAMUX makes it easy to:
- Inject your data into the associative memory
- Perform inference (a.k.a., “Memory Retrieval”, “Error correction”, or “the forward pass”) by autograd-computed gradient descent of the energy function!
- Build complex, powerful networks using arbitrary energy functions. E.g., we can easily build the Energy Transformer in this framework using a couple lines of code. See this tutorial (WIP).
We are continually trying to enrich our tutorials
, which are implemented as working Jupyter Notebooks. HAMUX is built on the amazing JAX and equinox
libraries.
How to Use
We can build a simple 4 layer HAM architecture using the following code
= (32,32,3) # E.g., CIFAR images
img_shape
class ConvSynapse(Synapse):
conv: tx.Module
def __init__(self, conv:tx.Module):
self.conv = conv
def energy(self, g1, g2):
if self.initializing():
= tx.next_key()
key = g1.shape[0]
features_in = g2.shape[0]
features_out self.conv = self.conv.init(key, g1)
return jnp.multiply(g2, self.conv(g1)).sum()
def DenseSynapse(eqx.Module):
W: jax.Array
def __init__(key: jax.Array,
int, # Dimension of input `g1` to the energy
dim1:int, # Dimension of input `g2` to the energy
dim2:
):self.W = 0.01 * jr.normal(key, (dim1, dim2))
def __call__(self, g1:jax.Array, g2:jax.Array):
return -jnp.einsum("...c,...d,cd->", g1, g2, self.W)
def ConvSynapse(eqx.Module):
W: jax.Array
def __init__(key: jax.Array,
int, # Dimension of input `g1` to the energy
dim1:int, # Dimension of input `g2` to the energy
dim2:
):self.W = 0.01 * jr.normal(key, (dim1, dim2))
def __call__(self, g1:jax.Array, g2:jax.Array):
return -jnp.einsum("...c,...d,cd->", g1, g2, self.W)
= {
neurons "image": hmx.Neurons(lagr_identity, img_shape),
"patch": hmx.Neurons(lagr_tanh, (11,11,16)),
"label": hmx.Neurons(lagr_softmax, (10,)),
"memory": hmx.Neurons(lagr_softmax, (25,))
}
= {
synapses "conv1": hmx.ConvSynapse((3,3), strides=3),
"dense1": hmx.DenseSynapse(),
"dense2": hmx.DenseSynapse(),
}
= [
connections "image","patch"], "conv1"),
(["label", "memory"], "dense1"),
(["", ""], "dense2"),
([ ]